The Internet of Things (IoT) a massive group of devices containing sensors or actuators associated together over wired or remote systems. As per the estimation, more than 25 billion devices will be associated by 2020; IoT has been exponentially growing over the previous decade. Amid the development, security has been distinguished as one of the weakest zones in IoT. While actualizing security inside the IoT network, there are a few challenges including heterogeneity along with the addition of a number of gadgets that should be tended to. To approach the difficulties in anchoring IoT devices, it is proposed to utilize machine learning inside an IoT gateway to help secure the framework. According to the research, utilizing Artificial Neural Networks in a gateway to recognize anomalies in the data sent from the edge gadgets, have henceforth proved that this methodology can enhance the security of IoT frameworks.
Creating strong privacy and security conventions for data sharing devices is critical, because the gadgets, applications, and communication networks become progressively integrated. Moreover, as these frameworks develop, cyber attacks on the IoT are ending up progressively intricate. The security arrangements important to battle these attacks are computationally substantial and many times include a huge correspondence load. Moreover, because of their computational capacities, numerous IoT devices are more vulnerable to attacks. For instance, the sensors utilized for second-rate security insurance, are often designed to be low cost. Because of their computational limitations, they might take a time or may have difficulty recognizing an early cyber attack.
Machine learning-based methodologies have been utilized to execute critical security frameworks, including IoT validation, IoT access control, the secure offloading, and last but not the least malware detection strategies. Actually, these machine-learning approaches frequently help to defeat the computational restrictions of IoT gadgets, improving their security while keeping up or diminishing their locally available computational necessities.
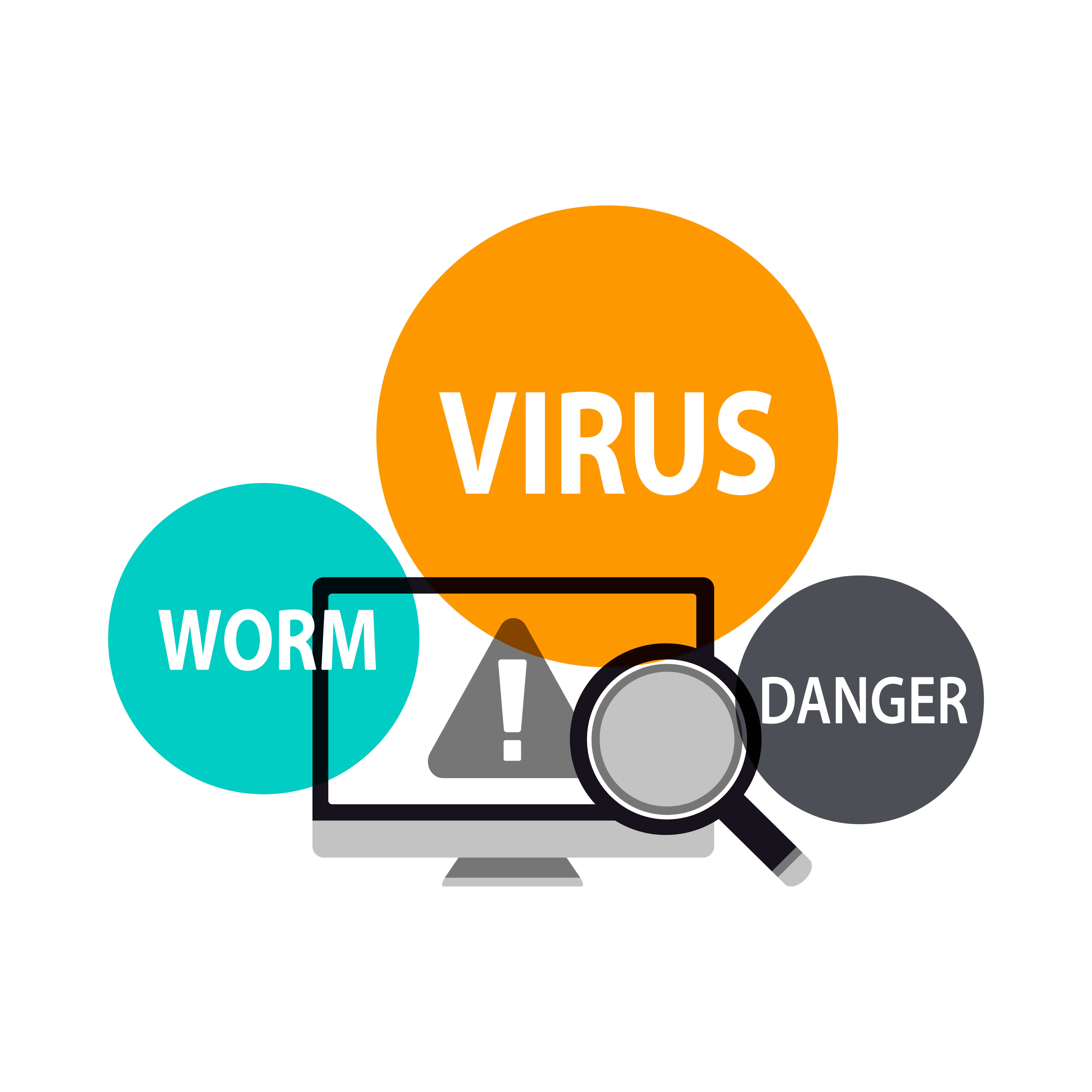
Illustration of virus detection
- Malware Detection
IoT gadgets must have the capacity to distinguish malware to avert dangers to security and execution. Supervised learning strategies can be utilized in IoT gadgets to recognize malware by distinguishing atypical behaviour. For instance, a malware detection anomaly utilizes k-nearest neighbours to cluster network traffic and after that utilizes random forests to distinguish malware among the traffic. With the end goal to constrain the load on the IoT gadget and to accomplish quicker computational speed, this malware detection can likewise be offloaded to a server.
To augment malware detection performance, Q-learning can choose the ideal edge for offloading to precision while limiting latency. Another technique is, the Dyna-Q method can likewise be utilized to locate the ideal offload limit. This strategy has been appeared to build accuracy and decline latency when contrasted with the Q-learning approach.
- Safe IoT offloading
With secure offloading, IoT gadgets can utilize internal, cloud-based computation and storage assets for tasks that require substantial computational power or for which latency must be limited. Additionally, to how Q-learning can be utilized to distinguish the ideal edge for IoT confirmation, Q-learning can be utilized to recognize the ideal rate of offloading data to battle sticking and ridiculing assaults.
In this situation, the Q-work decides the long haul reward of the offloading data rate dependent on power sticking the framework, the significance of the task, the channel data bandwidth, and the channel gain. Other machine learning systems can additionally enhance the performance. For instance, given enough computational power, a deep Q-network method can quicken the learning speed past that of Q-learning alone.
- Access Control
With access control, IoT gadgets prevent resource access by unapproved users. This procedure is made more difficult in IoT gadgets because networks are comprised of different kinds of data from an assortment of sources. Machine learning techniques like support vector machines, k-nearest algorithm, and neural systems have been utilized to distinguish unapproved users. Because of the intricacy of this kind of security, computational confinements regularly oblige the security of lower-grade IoT gadgets.
Access control conventions can be created utilizing machine learning procedures and afterwards executed in the IoT gadgets as a basic decision process. This method of overseeing access control decreases the vitality and computational power required by the framework, and, subsequently, builds the device’s lifespan. For instance, a k-closest neighbour strategy can be utilized to recognize anomalies among the information, giving a technique to distinguish any unauthorized user. Other machine learning procedures, for example, support vector machines, have been utilized to distinguish attacks on Internet activity and power networks.
- Authentication
One imperative security segment, verification, permits IoT gadgets to recognize between source hubs and outside attacks. With the end goal to keep up low utilization of computational power, verification strategies normally give security insurance by concentrating on the highlights of radio channels and transmitters in the physical layer (PHY) of the gadget. These PHY-layer attributes are then contrasted with the qualities distinguished by the transmitter. To decide if a transmission is authentic, the attributes are contrasted to a threshold, which is planned to maximize the precision of the classification. Since the environment of the IoT is erratic, notwithstanding, it tends to be troublesome for an IoT gadget to pick a suitable threshold to amplify this precision.
Securing IoT gadgets has turned out to be progressively troublesome as bigger amounts of data are being shared through the network utilizing moderately low-level innovation. Cyber attacks are winding up progressively unpredictable and are harder to battle, particularly when utilizing equipment with limited computational capacity. However, the security of user data stays critical with these threats.
The objective is to have the capacity to make the most utilization of all these associated gadgets that are finding their way into your home without agonizing over them keeping an eye on you, hurting you, or getting to be complicit in violations that will be submitted against you. Machine learning techniques are the key way to anchoring the IoT. Machine learning has demonstrated its value in a wide range of territories. Ideally, it will likewise help secure one fastest growing sectors in the tech industry.